What Is Inferential Statistics ? : An Overview
Updated: December 16, 2024
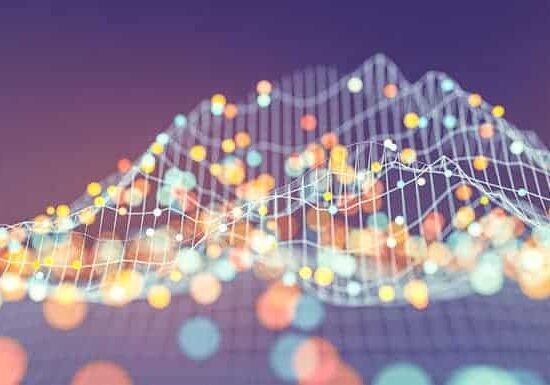
Ever wanted to know something specific about a group of people but felt overwhelmed by how much data you’d need to collect? Statistics and population parameters help people to understand raw data. There are different types of measurements that can be pulled from data. These measurements depend on the methods used to get data in the first place. Each methodology is used for its respective purpose. Here, we will answer the questions: “What is inferential statistics?” along with “What is the difference between descriptive and inferential statistics?”
What is Inferential Statistics?
Inferential statistics provides a way to draw conclusions about broad groups or populations based on a set of sample data. In some instances, it’s impossible to get data from an entire population or it’s too expensive. Inferential statistics solves this problem.
In order for inferential statistics to accurately reflect a population on the whole, the sampling methods need to be unbiased and random. To obtain an overview of a sample, you can use a statistic. The most common kinds of estimation are the interval estimate and point estimate. Point estimate deals with parameters and may be something like the sample mean. An interval estimate is a range of values and can be the confidence interval, for example.
Since a sample is just a portion of a broader set of data points, it could contain error. This is known as the sampling error. The sampling error is the difference between sample values (statistics) and population values (parameters).
What is Descriptive Statistics?
When you’re looking to describe or create a narrative based on data analysis, then descriptive statistics come into play. Descriptive statistics offer a way to summarize data. This can happen by way of noticing patterns and trends in data or using the information at hand to assess hypotheses that exist.
When there is a lot of data, it can be hard to visualize what the data is saying. Descriptive statistics makes it easier to interpret data. In order to do so, descriptive statistics will cover:
- Measures of central tendency: This is how you can pinpoint the central position of a frequency distribution. The central position can be determined by using the mode, median, and mean. The mean is an average, whereby you take all values, add them up, and divide by the total number of values presented. The mode is the most commonly recurring value. The median is the value in the middle from smallest to largest.
- Measures of spread: Measures of spread describe how spread out the values are. It helps to understand how large or small the range of values is within the data. These statistics include: range, absolute deviation, standard deviation, variance, and quartiles.
What is the Difference Between Descriptive and Inferential Statistics?
As mentioned in the introduction, descriptive and inferential statistics each serve their own purpose. While descriptive statistics are a way to review exact numbers, inferential statistics allows for generalizations to be made.
Rather than taking a sample and applying it to a whole population as a specific number, inferential statistics provides conclusions and generalizations. Since there isn’t a way to get exact numbers, scientists and researchers must also assess how accurate these inferences are through numbers like confidence intervals and other parameters.
Related Key Terms
To further understand inferential and descriptive statistics, let’s review a few key terms that appear in each discipline:
- Population parameters: Population parameters are aspects that describe groups or populations. A population is a way to describe all members of a group. A sample is a subset of the population. For example, if the population you are studying is how many customers made a purchase at a store, then a population parameter may be that 50% ordered online.
- Sampling error: The sampling error refers to the difference between a population parameter and the sample statistic that is used to measure it.
- Sample statistics: A sample statistic is information you get from a sample. To exemplify, you may want to know how many college students in America are also parents. Rather than asking every single student in the country if they are a parent, you can take a sample of the whole population by surveying 1,000 random college students. Then, you can gain the knowledge of whether they are parents or not and conclude the statistic that roughly 26% of undergraduates are parents.
- Confidence intervals: A confidence interval is the probability that a population parameter will be within a set of values for a specified proportion of times it’s pulled. It’s a way to measure how certain or uncertain a sampling method is.
- Hypothesis testing: Hypothesis testing allows analysts to test their assumptions (hypotheses) based on a population parameter.
Inferential Statistics in Action
The ability to access data is becoming increasingly easier with time. The combination of technology and computer science allows for a mass amount of data to be translated into valuable insights. Statistics allows businesses and people to make better decisions and know more about the state of the world.
If you’re interested in working within the field of business or computer science, then inferential statistics will play a part in your life. For those who wish to learn more about data science, then a degree in computer science is a great start. At University of the People (UoPeople), we offer tuition-free computer science programs that can open the door to many career opportunities.
The Bottom Line
Inferential statistics makes it possible to learn a lot about entire populations by utilizing information gained from a random sample. This method is valuable across many fields, including: computer science, business, healthcare, public policy, financial policy, and much more.